In our fast-paced digital world, leveraging data effectively is no longer just a luxury; it is essential for staying competitive. Businesses are flooded with vast amounts of information, but the real value comes from how we analyze and interpret this data. Turning raw data into actionable insights can significantly enhance decision-making processes, leading to smarter strategies and better outcomes.
Understanding the Value of Data Analytics
Data analytics involves both qualitative and quantitative methods used to improve productivity and drive business success. In simple terms, it's about applying statistical methods and algorithms to find patterns and insights within data.
When executed effectively, data analytics not only helps businesses assess their past performance but also equips them to predict future trends. This capability is crucial in uncertain and rapidly evolving markets.
When organizations transform data into strategic insights, they can respond quickly to market changes. Businesses that excel in data analysis can anticipate shifts and modify their strategies accordingly. For example, companies like Amazon use data analytics to analyze customer purchasing patterns, which helps them recommend products effectively and increase sales by over 30%.
The Role of Big Data in Decision-Making
Big Data includes various data types—structured, semi-structured, and unstructured—gathered from sources such as social media, transactional records, customer interactions, and IoT devices. Analyzing Big Data allows companies to extract insights that lead to informed decisions.
Big Data is often described by the "Three Vs": Volume, Velocity, and Variety. Organizations accumulate vast amounts of data rapidly from diverse sources. For instance, Facebook processes about 100 petabytes of data daily. Being able to sift through and analyze such massive datasets is where effective tools and technologies come into play.
Tools for Analyzing Data
Utilizing a range of tools is crucial for effective data analytics. Some key tools that can significantly boost analytics capabilities include:
Python and R
Python and R are two powerful programming languages favored by data scientists for their versatility and expansive libraries. For example, Python's Pandas library helps with data manipulation, while Matplotlib provides robust visualization options. This allows users to perform thorough data analysis.
R, on the other hand, is particularly useful for statistical analysis and graphical representation, appealing greatly to academic and industry researchers. Familiarity with these languages can empower analysts to generate more accurate insights.
SQL Databases
Structured Query Language (SQL) databases are vital for managing and querying relational databases. Proficiency in SQL is necessary for data analysts, as it enables them to efficiently retrieve and manipulate data.
This expertise ensures timely access to the right data, leading to faster insights and more responsive decision-making.
Data Visualization Software
Tools such as Power BI, Tableau, and Excel are critical in communicating data insights. By providing visual representations of data, organizations can effectively share insights with stakeholders, making data-driven decisions more tangible.
Visualizations simplify complex data and highlight trends, anomalies, and patterns that might otherwise be overlooked. Research indicates that visual data can increase understanding and retention by up to 80%.
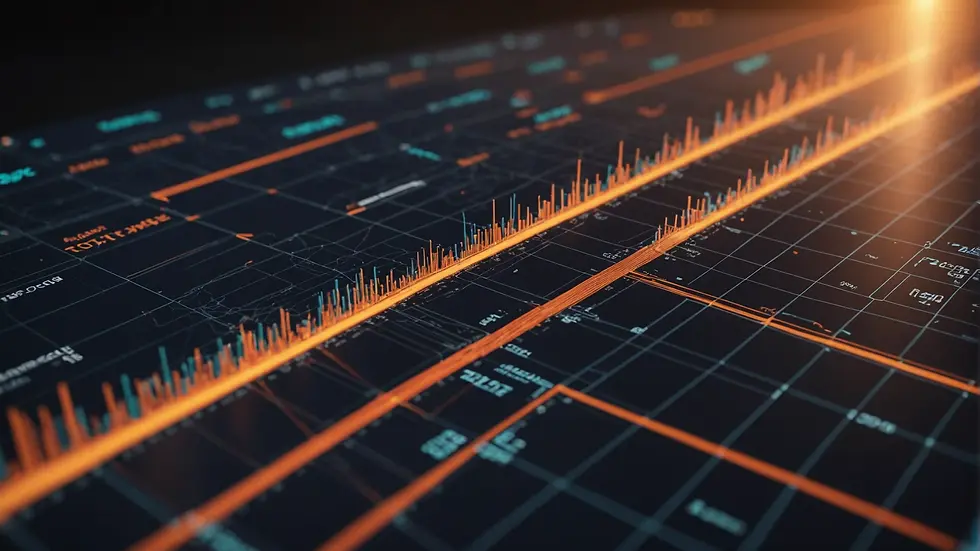
The Importance of Machine Learning in Analytics
Machine learning, a branch of artificial intelligence, allows systems to learn from data, identify patterns, and make decisions with minimal human input. By integrating machine learning into data analytics, organizations greatly enhance their ability to make predictions based on historical data.
For example, predictive analytics in retail uses machine learning algorithms to forecast consumer purchasing behavior. A well-known retailer reported a 15% increase in sales by utilizing such predictive models to anticipate customer needs.
Transforming Raw Data into Insights
The journey from raw data to meaningful insights involves essential steps:
Data Collection
The first step in analytics is obtaining relevant data. This can include data from internal sources like CRM systems, sales databases, and inventory management tools, along with external data such as market research or social media trends.
Data Cleaning and Preparation
Once collected, data must be cleaned and prepared for analysis. This means removing inconsistencies, addressing missing values, and formatting data correctly. Proper preparation is crucial for ensuring accurate analysis results.
Data Analysis
After preparation, the next step is analyzing the clean data using statistical methods, algorithms, and visualization tools. Analysts leverage programming languages like Python or R along with SQL skills to extract valuable insights.
Informed Decision-Making: Best Practices
When using data analytics for decision-making, consider these best practices:
Define Clear Objectives
Before beginning any analysis, outline specific insights needed and their purpose. This understanding will guide the analysis and ensure it aligns with organizational goals.
Utilize A/B Testing
To validate new strategies or changes, implement A/B testing to compare performance metrics. This approach allows businesses to base their decisions on real-world data rather than guesswork.
Continuously Monitor and Adapt
Analytics is an ongoing process. Use monitoring tools to evaluate data performance and adapt strategies based on newly emerging trends and insights.
Cultivate a Data-Driven Culture
Encourage a culture where data-driven decision-making is valued at all organizational levels. Training staff in data literacy and providing access to analytical tools can elevate overall decision-making effectiveness.
Overcoming Challenges in Data Analytics
Despite the clear benefits of data analytics, organizations may encounter several obstacles during implementation:
Data Privacy Regulations
With regulations like GDPR gaining traction, organizations must comply with privacy standards while still extracting insights from data. Creating comprehensive data governance policies can address these concerns.
Integrating Data from Various Sources
Integrating data from multiple, disconnected sources can pose a significant challenge. Developing a unified data architecture can ensure smoother data flows and more precise insights.
Skills Gap in Data Science
There is an ongoing demand for skilled professionals who can analyze and interpret complex data. Investing in training and development can help bridge this skills gap, allowing teams to maximize their analytics potential.
The Future of Data Analytics
As technology advances, data analytics is becoming progressively sophisticated. The emergence of artificial intelligence, machine learning, and automation is set to change how data is processed and analyzed.
Organizations that adopt these technologies may unlock even deeper insights, fostering innovation and informed decision-making across different sectors.
Unlocking Insights for Informed Decision-Making
Transforming data into actionable insights is a journey every organization must undertake. With the right tools, clear strategies, and a commitment to analysis, businesses can extract valuable insights that genuinely inform their decisions.
As data volumes continue to surge, understanding how to analyze and leverage it will be crucial. By embracing data analytics, organizations can drive more informed outcomes and equip themselves to thrive in today’s dynamic landscape.
Those willing to explore the potential of data analytics will find themselves better equipped to navigate the complexities of modern decision-making. By prioritizing analytics, organizations can access a wealth of insights that not only enhance strategic decisions but also foster innovation and growth.
Comments